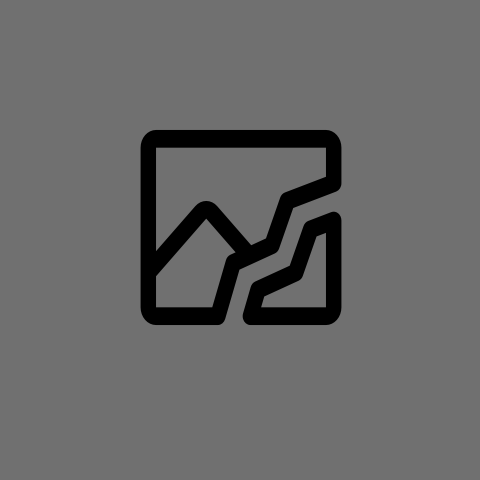
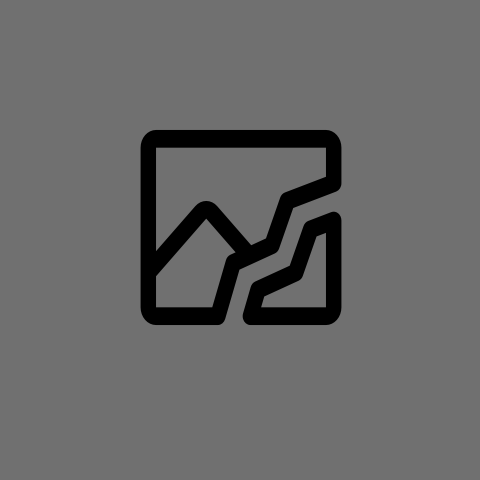
Interesting, is Partner in Crime (PIC) like an open source co-pilot type project? I haven’t heard of it before (did you coin this phrase yourself, or is it well known)?
I ask because the tasks you describe (json/md/function calling/empathy) and then the name itself, all basically make it sound like the “open source” models equivalent of a co-pilot model.
Slightly off-topic – I’ve been testing 13b and 7b models for awhile now… and I’m really interested if people have a good one to check out, because at least for now, I’ve settled on a 7b model that seems to work better than most other 13b models I’ve tried.
Specifically, I’ve been using OpenChat 3.5 7b (Q8 and Q4) and it’s been really good for my work so far, and punching much higher than it’s current weight class… – Much better than any of the 13b models I’ve tried. (I’m not doing any specific tests, it just seems to understand what I want better than others I’ve tried. – I’m not doing any function calling but even the 4bit 7b model is able to generate JSON as well as respond coherently.)
Note: specically using the original (non-16k) models; the 16k models seem to be borked or something?
Link: https://huggingface.co/TheBloke/openchat_3.5-GGUF